Cultivating the Future: How Data Science Revolutionises Agriculture
- Group Digital
- Jul 27, 2023
- 2 min read
Updated: Jun 6, 2024
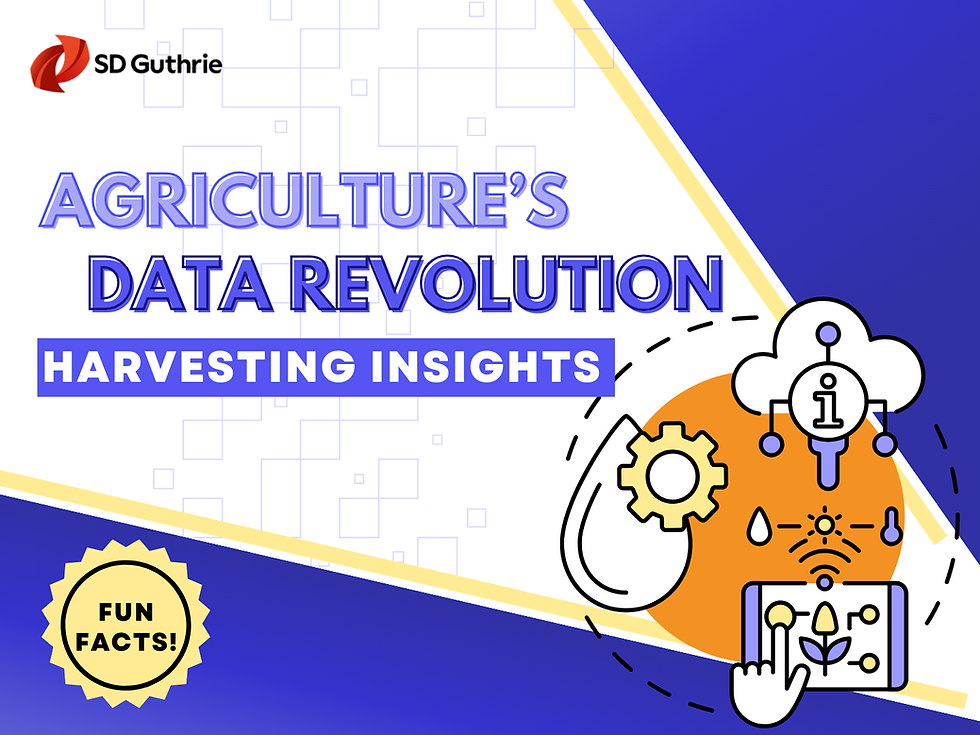
Data science can be used in agriculture to improve various aspects of the industry, including crop yields, resource management, and decision-making.
Here are a few examples of how data science is being used in agriculture:
Predictive Modeling:
Data science can be used to create predictive models that can help farmers forecast crop yields, optimise irrigation schedules, and identify potential pests and diseases.
Precision Agriculture:
Data science can be used to support precision agriculture, which involves using sensors, drones, and other technologies to gather data on soil conditions, weather patterns, and other factors that can affect crop growth. This data can be used to create customised recommendations for farmers, such as optimal planting and fertilisation strategies.
Supply Chain Optimisation:
Data science can be used to analyse data from the agriculture supply chain, including data on production, transportation, and distribution. This can help identify bottlenecks and inefficiencies in the supply chain, leading to more efficient and cost-effective operations.
Food Safety:
Data science can be used to analyse data on foodborne illness outbreaks and identify potential risk factors, such as specific types of food or production practices. This can help farmers and food processors implement more effective food safety protocols to reduce the risk of outbreaks.
Data Science: Improving Predictive Modeling in Agriculture and Yield Prediction?
Predictive modeling in agriculture involves using data science techniques to create models that can predict future crop yields based on historical data and other relevant factors. Here's an example of how this might work:
1. Collect Data
The first step in creating a predictive model for crop yields is to gather a large amount of data on various factors that can affect crop growth, such as soil conditions, weather patterns, and irrigation schedules. This data can be collected using sensors, drones, and other technologies.
2. Clean and Prepare the Data
Next, the data needs to be cleaned and prepared for analysis. This involves identifying and removing any errors or inconsistencies in the data, as well as selecting the relevant features that will be used to build the model.
3. Build the Model
Once the data is ready, a machine learning algorithm can be used to build a predictive model. This typically involves training the model on a portion of the data, and then testing it on the remaining data to evaluate its accuracy.
4. Evaluate and Fine-Tune the Model
The accuracy of the model can then be evaluated and fine-tuned by adjusting the model's parametres or by adding additional data. This process can be repeated until the model is able to make accurate predictions of crop yields.
By using data science to build predictive models, farmers can more accurately forecast crop yields and make informed decisions about how to optimise their operations. This can help them increase yields, reduce costs, and improve the overall efficiency of their farms.
Source:
DTN by DTN Team
Comments